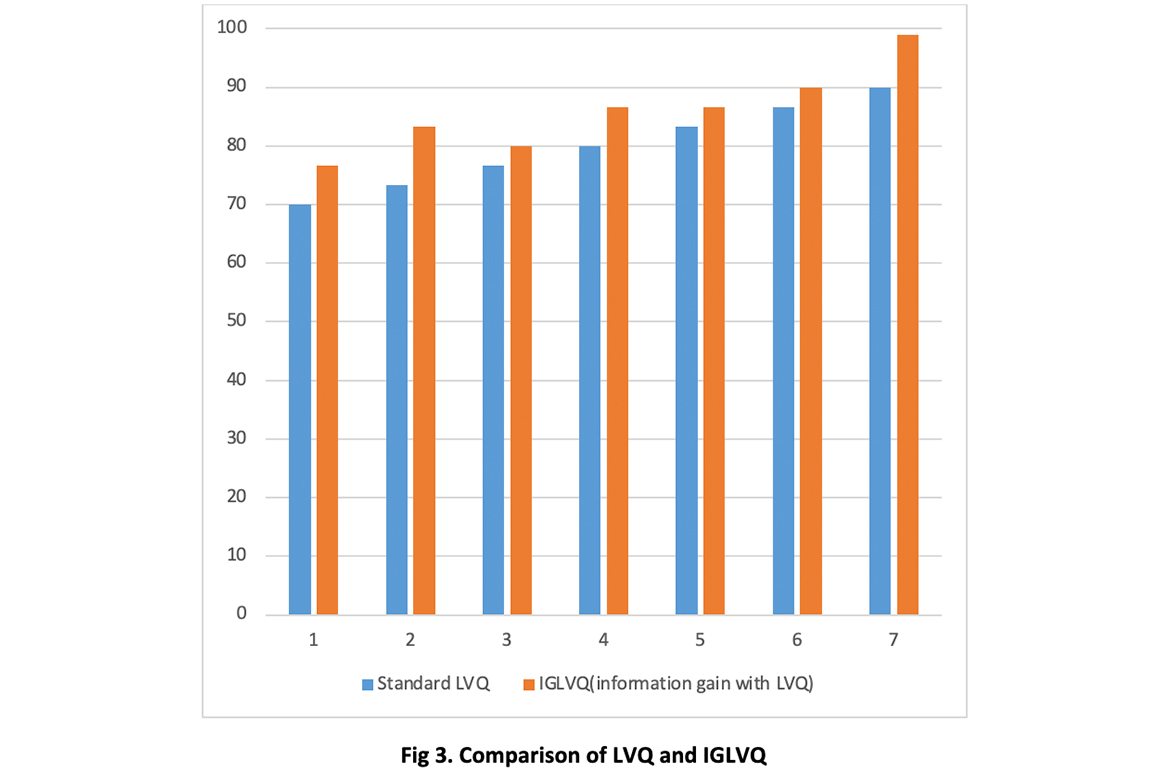
ABSTRACT: In this paper, an Information Gain based Weighted Linear Vector Quantization (IG-WLVQ) is applied to the heart dataset available in UCI machine learning repository for the prediction of heart disease. It considers all attributes of the data set. The IG-WLVQ method weights the attributes according to the information gain while training the dataset. It is found that the classification accuracy approaches to 98.9%.
Keywords: LVQ, IG-WLVQ, ML
Introduction
The mortality rate due to heart disease is increasing day by day in human beings. It is a matter of serious concern worldwide. Therefore, effective measures are highly essential to control the disease. Machine learning techniques have already proven as the most reliable and perfect platform for health care sector. The Cleveland heart dataset of UCI machine repository is considered for this analysis. Most of the researchers considered 13 attributes and one class level of Cleveland heart dataset neglecting the significance of remaining attributes [1][2]. The Majority of research which was carried out with those attributes provides good accuracy due to small data set. Some of the authors use ensemble technique for feature extraction to improve classification accuracy [3][4]. In this paper, an attempt has been made to use the whole dataset without any information loss. Our unique purpose is to use all attributes which is applied to LVQ [5]. This must be accomplished by placing information gain along with LVQ algorithm such that the classifier performance is boosted recognizing it as a good classifier.